How businesses use analytics to learn about their customers and find new ones
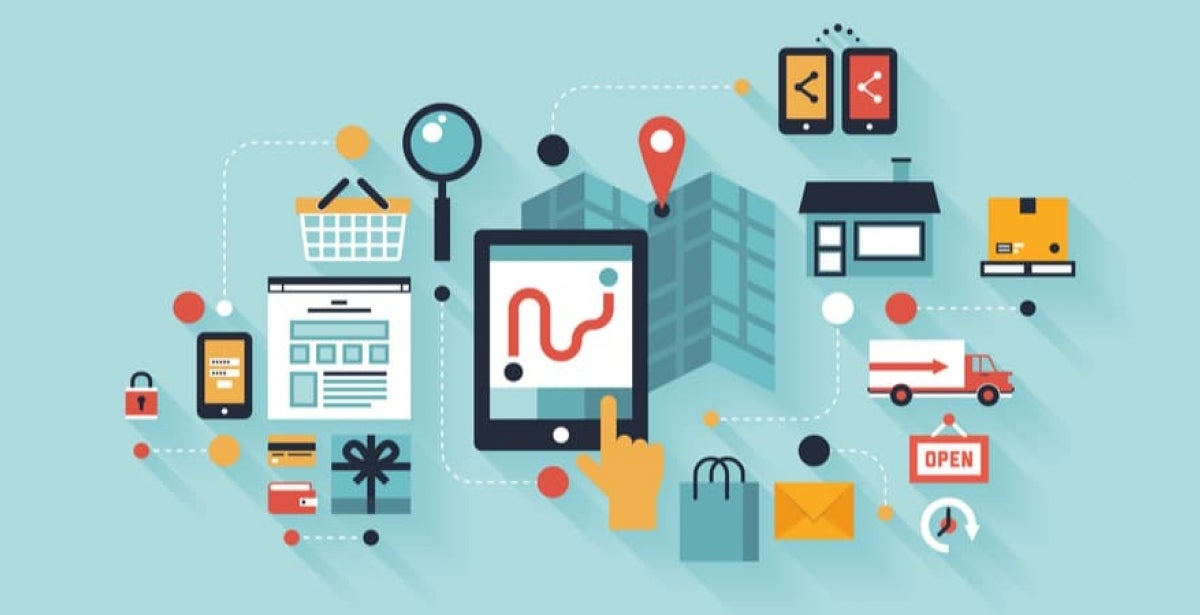
Marketing departments in Australia and around the world are increasingly relying on the power of data science to capture consumer attention. While the creation of advertisements still relies on human creativity, tasks such as ad purchasing and customer targeting are data-driven and can be made more efficient by analytics and machine learning. According to McKinsey, data science could soon create USD $8.6 trillion in annual value for the global marketing industry, a whopping 40 per cent of the $21.2 trillion of what it will create for the economy as a whole.
The data science/marketing relationship in a nutshell
Marketers can make effective use of data science due to the large amount of data available to them. The retail market is characterised by a high number and frequency of interactions between retailers and consumers (e.g., sales, website visits), all of which provide data. This gives marketers unique access to large, up-to-date datasets that can be used to discover insights into consumer behaviour or to automate certain marketing services.
Modern businesses interact with customers through multiple channels, including retail stores, online and offline advertisements, first-party online sales and third-party online sales (e.g., Amazon). To achieve a unified view of these interactions, businesses rely on data scientists to integrate the data produced by each channel into a unified whole. Through this process, data scientists can gain insights into the overall performance of multi-channel ad campaigns and learn why some channels produce better results than others.
Data science - use cases for marketing
Data science permeates nearly every aspect of the marketing industry; however, the majority of the value of data science comes from analytics-driven services, which enable advertisers to evaluate the effectiveness of their campaigns, target audiences with precision, and automate the purchasing and placement of digital advertisements.
Measuring return on investment (ROI)
Before the advent of data science, companies had limited ability to measure the performance of their marketing efforts. Marketers were forced to rely upon a few basic data points, such as customer surveys and sales trends, to evaluate the success of their campaigns. This made it difficult for firms to make cost/benefit-driven decisions about their marketing direction, which meant that marketing was often viewed more as a cost of doing business than as an engine to create value and drive growth.
The corporate world was quick to see analytics as an opportunity to fix this issue, and so marketing departments were among the first to face executive pressure to embrace data-driven decision making. Increased access to data provides businesses with the ability to measure campaign-relevant data in fine detail, which in turn provides them with the ability to quantify the effectiveness of their campaigns. This process relies on data scientists to assess and improve the quality of their firm’s marketing data, and then to develop analytics models capable of producing insights which achieve statistical significance.
Thanks to data science, modern advertising campaigns can now be evaluated according to a large variety of key performance indicators (KPIs)—metrics which are found within campaign data. Modelling the relationships between these KPIs enables data scientists to discover precise insights into the factors that affect how an ad performs with different audiences, such as location, age, gender, etc. Specific emotional reactions—how audiences feel about a brand after seeing an ad—can also be measured using these methods.
Put simply: Data scientists do not just prepare a model and then hand the results to the marketers. They are vital to the practice of interpreting the results of their analyses.
By evaluating campaign performance criteria against data which describes the content of the ads (i.e., video/image, colours, subject matter, etc), data scientists can learn what type of content appeals to specific types of people. This understanding empowers marketers to tailor ad campaigns to target consumers at the individual-level, a type of marketing called “personalised marketing”.
Personalised marketing & audience segmentation
Personalised marketing, also called 1:1 marketing, is a type of marketing designed to appeal to the specific individual. Its goal is to present each individual with the right ad, at the right place, at the right time, in order to make a sale. Because personalised marketing relies on being able to advertise directly to individual consumers, it’s mostly used on online and mobile platforms.
The use of predictive analytics is vital to the effectiveness of personalised marketing. In order to ensure that their efforts are accurate, data scientists use existing customer data to train machine learning models to understand how various consumer traits change the likelihood that an advertisement will lead to a sale. A large amount of data is necessary to train predictive models for personalised marketing; however, once trained, many models are able to work effectively without actually having much data on the individuals they are being used to market to.
Certain advertising platforms, such as Facebook and Google, possess large amounts of personal data that can be used to predict what ads (purchased by a third-party) should be shown to an individual. The ability to provide highly-targeted marketing services is part of why these platforms are so valuable to advertisers. Due to the statistical complexity required to develop a predictive model based on a large number of customer features, these platforms’ rely on highly sophisticated models to power their marketing services.
Intelligent and automated ad purchasing
Many marketing campaigns have multiple performance targets, such as key demographic targets, customer acquisition targets, and channel-specific targets. In order to figure out the optimum “marketing mix” to hit these targets, data scientists use machine learning on past data to predict the results of future campaigns. These predictions can provide marketers with insights into where they should purchase ads and how much they will need to spend to achieve their goals.
The use of machine learning to predict where, when, and how much advertising to buy allows marketers to automate much of their ad purchasing. Most digital advertising already relies on a “real-time bidding” system in which ads shown to individual website visitors/app users are purchased automatically by specialised marketing software. Incorporating data science into this automated process (which is called “programmatic advertising”) enables marketers to leverage the efficiency of automation without the costs of bulk purchasing ads at a low rate of success.
In addition to improving campaign success, the use of automated advertisements also enables the collection of rich data about the ads themselves. For example, many marketers use programmatic marketing to compare new advertising content to their current ad content by using analytics to view how each ad performs when shown to different consumer segments. This ability to gather granular data on comparative ad performance is a key part of why firms expect marketers to use analytics to continuously improve the results of their campaigns.
Data scientists that work in marketing are part of the leading edge of their profession. Driven by their use of data science, Chief Marketing Officers now spend more on IT than Chief Information Officers, and marketing departments are gaining influence over how their companies use technology. Analysts expect this upward trend to continue; a 2018 Forrester analysis predicts that firms will spend USD $23 billion annually on marketing automation by 2023, an over 100 per cent increase from the $11.4b spent in 2017.
The opportunities for data scientists in the marketing world are growing. The online Master of Data Science at the University of New South Wales Sydney provides the education necessary to start a career in the field. The flexible as little as two years program focuses on the statistics and machine learning methods that marketers are looking for. Completion time dependent on individual study path, RPL, leave and course availability. Please speak to a Student Advisor for more information.
UNSW’s career-focused data science programs provide the job-ready skills necessary to make your goals a reality. Get in touch with our enrolment team at 1300 974 990.